Decoding the promise and peril of large language models
Matt Minshall takes a look at how new tools such as ChatGPT will revolutionise the world (or not).
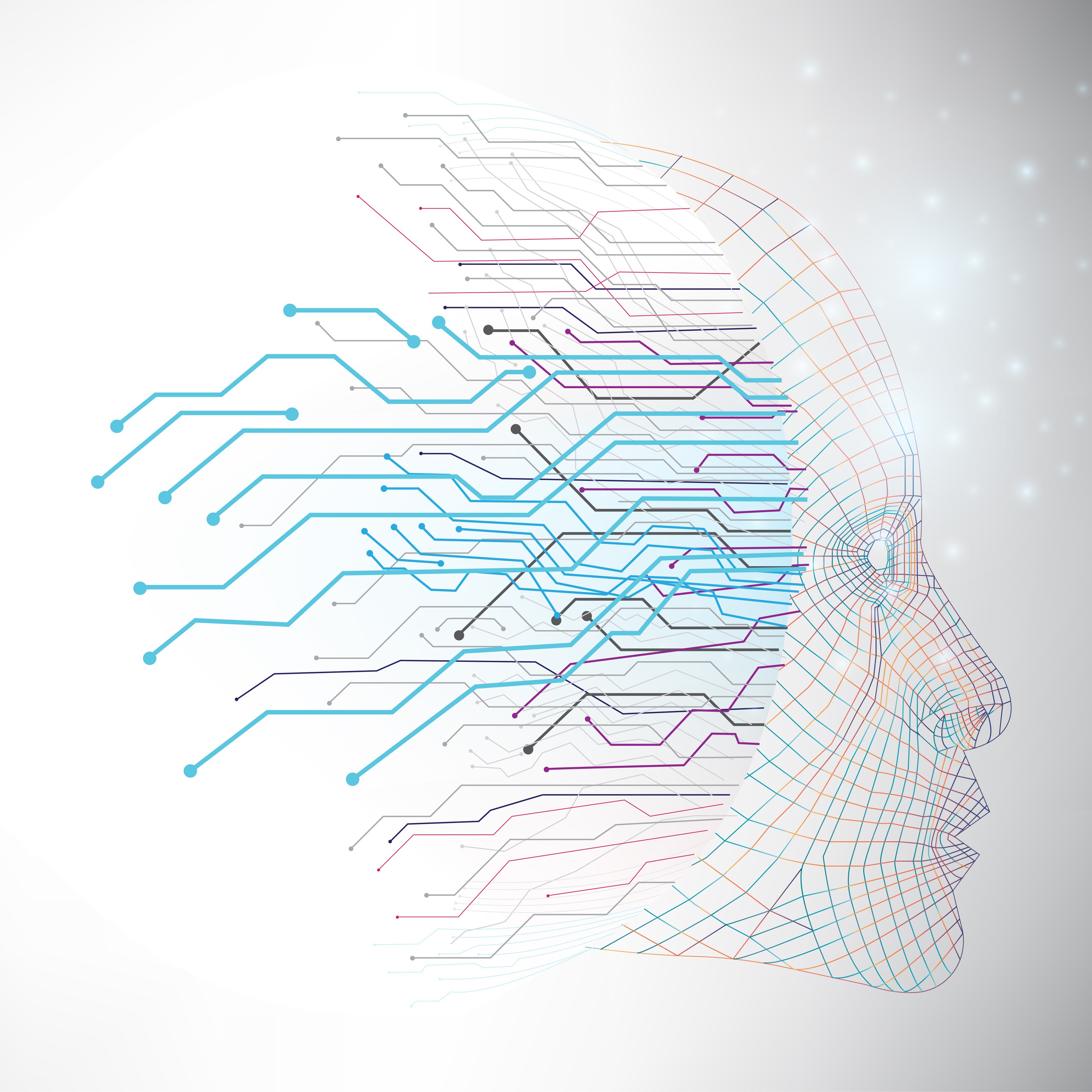
Adobe Stock | CNStock
Whenever a potentially game-changing new technology emerges, there is an explosion of reactions. But unless they are as radical as antibiotics, steel, or the telegraph, concepts that appear novel are rarely entirely new, and the impact is rarely as good or as bad as at first it may appear.
Some of the latest discussions, euphoria, and fears revolve around the impact of Large Language Models (LLMs), which have become of keen interest. LLMs are AI-powered systems that are trained on vast amounts of data, allowing them to generate human-like text and understand complex language patterns. LLMs are not new, but the app that has brought the technology into the popular space is ChatGPT, designed and owned by Elon Musk’s OpenAI. GPT stands for "generative pre-trained transformer," which is a programme that can realistically write like a human. GPTs have been around for several years, and although they are growing in popularity, their use has become a dilemma across all fields.
Most new technology has both pros and cons, as well as people who like it and people who don't.The abacus has been around since ancient Babylon. It is one of the earliest known machines that helped people, and it is likely that it had its critics. The use of pocket calculators for exams was initially strongly opposed, but has evolved and is now commonplace, despite the fact that some people still object to such an application.
This blog explores the good, the bad, and the ugly of LLMs. The good is the benefit to humanity; the bad is the negativity; and the ugly is the misuse and abuse.
The good
LLMs are revolutionising the field of natural language processing, making it easier for people to communicate with machines, and this is a good thing. LLMs can understand complex language patterns and generate text similar to that of humans, which has opened up new possibilities for chatbots and virtual assistants to make them more user-friendly.
They are also capable of processing large amounts of data and can be used to personalise content for individual users. They can deal with much of the drudgery of standard human text generation for routine tasks, which can save time and money for businesses, allowing them to focus elsewhere.
The bad
If the data used to train an LLM is biassed, then the model will also be biassed. This allows them to perpetuate and amplify existing biases, such as racial, gender, and other prejudices, which can have deeply negative impacts on individuals and communities.
I asked ChatGPT to write a 50-word poem about the leader of a political party of a government in power, and it produced a bland but perfectly structured verse praising the person in question. When asking the same question about the leader of the opposing party, the response was as follows:
As an AI language model, I do not promote or endorse any political figures or parties. My purpose is to provide helpful and informative responses to your questions without bias.
…quod erat demonstrandum.
The vast amounts of data needed for LLMs often contain personal information, which can pose a threat to privacy. These models produce text that is remarkably similar to human text, which can make it challenging to distinguish between different types of content and cause management and accountability issues.
Detractors do not necessarily fear the technology but its impact: what effect might it have on society, the economy, or themselves? And this is not new; in 1492, a leading scholar, Johannes Trithemius, predicted that the printing press would never last. In his essay "In Praise of Scribes," he argued that handwriting was morally superior to mechanical printing - an opinion surely influenced by the fact that monks working as scribes worried that the printing press would put them out of work.
Much later, the term "Luddite" evolved and is now a blanket term used to describe people who dislike new technology. Its origins date back to an early 19th-century workers' movement that railed against the ways that mechanised manufactures and their unskilled workers undermined the skilled craftsmen of the day.
Another fear of the rise and accessibility of LLMs is that it will make educational assessment increasingly difficult. Students already use ChatGPT to do their work, and the traditional plagiarism checkers, tutors, and many apps are having trouble keeping up.
The ugly
The ugly parts include the misuse of weapons in warfare, the exploitation by criminals and terrorists, and the focus on commercial gain rather than the benefit of mankind; but these are sadly part of the world in which we live.
A deep concern is that as LLMs grow in capability, the need for human learning and the retention of knowledge may diminish. If the human mind loses analytical aptitude, the information programmed into LLMs will decline in value, resulting in a downward spiral into general ineptitude.
Every action has an equal and opposite reaction.
No doubt, skilled weavers made redundant by machines found work in industries making the very contraptions they once feared. The students who gleefully copied and pasted large chunks of internet script into their essays were caught out by plagiarism checkers, so the creation of LLMs accelerates the counterbalance of AI to differentiate man from machine. For academia, if students are unable to refer their arguments to authoritative reference, it may herald the welcome expansion of the intimacy of F2F evaluation via the viva!
When Open AI released ChatGPT to the public for free, it caused a stir, and students and writers all over the world identified the idea of getting AI to write their essays, dissertations, and other assignments. Edward Tian, a researcher at Princeton, reacted swiftly. His main concern was how it would affect employment and how it might disrupt education systems. What would be the point of learning to write essays when AI can effectively manage this with minimal effort? In a very short time, he created a new app, GPTZero. The app, which paradoxically uses ChatGPT against itself, monitors how much the AI is used.
Increasingly sophisticated AI is an inexorable part of the future but presents quandaries. If children no longer need to develop critical thinking skills, will humanity become simply unthinking mammals largely dependent on IT for much of their modus operandi, from basic living to global management? Are fictional films such as The Matrix and Ex Machina portents of the future? Probably not, but the race to ensure the ideal outcome, which is value for good while avoiding any devaluation, must be an enduring one.
Are LLMs any different from a pocket calculator? In principle, no; their answers may be multifarious, but they only answer the questions given by taking relevant keyword text and placing it into human-like patterns. Is their use any different from that of a pocket calculator? Yes, dramatically so, and this has good, bad, and ugly aspects. They offer numerous benefits, such as improved communication, personalization, efficiency, and education. But they have many drawbacks, such as bias, privacy concerns, and accountability issues, and the potential for misuse and abuse is apparent. To ensure the good of this dynamic new technology and others, a first step must be to outthink and outpace the negativity by considering all the implications of current and future capability and to extend the original Laws of Robotics to include all that the future will bring.
Note: The author used ChatGPT to generate parts of the text of this blog. In general, the results were clearly structured but bland and often repetitive. LLMs have no emotion and cannot give opinions, so any analysis offered – for or against – is generally simply tit for tat. The results were checked by GPTZero, which identified a high probability of machine generation in all cases and offered explanations of the indicators.